Reanalysis
Reanalysis is a method used to obtain a comprehensive picture of the state of the Earth system. This method involves combining the results from a weather forecasting model with observations to produce, generally for the entire globe, archives of a large number of variables representing atmospheric, oceanic and land surface conditions at increasingly fine scales with a temporal resolution of a few hours to several decades. Data sets produced in this way are known as reanalyses.
For anyone interested in the climate, reanalyses spanning several decades are invaluable: based on this series of portraits of the real atmosphere, the actual climate characteristics can be derived for a large number of variables.
Reanalyses are produced in much the same way as the analyses used to initialize a weather forecasting model when the time comes to generate a new forecast. However, over the years, more and more efficient weather forecasting models, sometimes very different from each other, have been developed as corrections have been made, the horizontal and vertical resolutions have been refined, and some variables have disappeared while new ones have been introduced.
As a result, the analyses lack homogeneity, making them difficult to use for studying the climate over long periods of time.
To remedy this uniformity problem, some forecasting centres have decided to revisit their archives in order to produce reanalyses. To do this, these centres select the most recent version of their weather forecasting model and data assimilation algorithm, and then set a horizontal and vertical resolution that will remain uniform throughout the reanalyzed period. New sources of satellite or radar observations can even be integrated into the procedure, improving the representation of the climate. This work is generally carried out over several decades corresponding to periods when observation networks are varied, dense and reliable.
Here are the main stages in the production of analyses and reanalyses:
1. Run the assimilation cycle on the observed data
The aim of this procedure is to integrate observations from various sources and regions of the globe (from upper-air sounding, surface weather stations, satellites, radar, etc.) into the weather forecasting model*. These observations represent the state of the atmosphere and the surface at the time identified as the initial time of the weather forecast to be produced.
The assimilation of observed data relies on sophisticated mathematical techniques that have to handle the fact that observations are made at different times and contain errors or are incomplete. This data can also come from a variety of sources and instruments with different spatial and temporal coverage. In fact, data is non-existent for several variables in a weather forecasting model.
*Like climate models, weather forecasting models are physical models based on the equations of fluid mechanics. These equations give them the ability to produce numerous variables that are consistent with each other in space and time.
2. Produce the analysis using the assimilated data
Once its assimilation cycle has been completed, the forecasting model establishes the most accurate snapshot possible of the atmosphere at the initial time for several dozen consistent variables on its computational grid, including variables that are not measured. This is what we call an analysis. In other words, analyses include two types of variables: (1) those for which the model has been able to take observations into account, and (2) those that are purely products of the model because no observations are available.
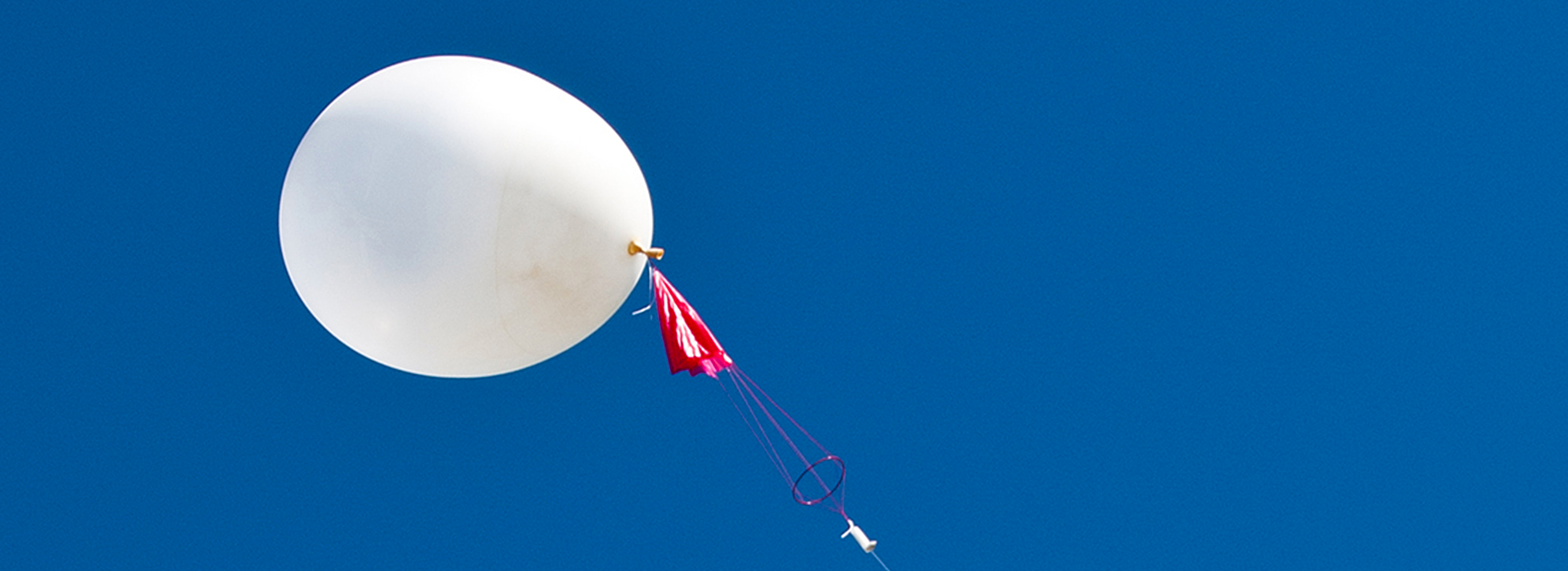
When the analysis is ready, the model uses it to initialize the simulation that will enable it to produce weather forecasts for the future. Analyses are produced two to four times a day and are stored by the various weather forecasting centres around the world.
Producing reanalyses requires a colossal amount of financial and technical resources, which are accessible to only a few major forecasting centres. The best known are the European Centre for Medium-Range Weather Forecasts (ECMWF; the ERA5, ERA15, ERA40, ERA-Interim reanalyses), the National Centers for Environmental Prediction (NCEP; the NCEP, NCEP-DOE Reanalysis II, CFSR and NARR reanalyses), NASA (the MERRA2 and MERRA reanalyses), the Japan Meteorological Agency (JMA; the JRA-3Q, JRA-25 and JRA-55 reanalyses), and the National Oceanic and Atmospheric Administration (NOAA; the 20th Century Reanalysis V3). In recent years, some centres have begun to produce reanalyses that include variables associated with the Earth’s surface (e.g. the ECMWF’s ERA5-Land and ECCC’s Canadian Surface Reanalysis V3).
Although reanalyses incorporate observed data and offer a fairly credible picture of reality, there are significant differences between the reanalyses by different forecasting centres. Here are the main reasons:
-
Disparities are greater in regions of the world where observations are rarer and more scattered.
-
To produce their reanalyses, the various forecasting centres use different weather forecasting models and different assimilation schemes.
-
The spatial resolution and the choice of observations vary.
-
Variables for which there are no observations potentially have the greatest disparities, as they are determined solely by the forecasting model.
Despite these uncertainties, reanalyses are a valuable and indispensable tool for climate science, as they enable analyses of many variables and their interactions. They are used to improve our understanding of various hazards. They are also used to validate climate models (ESMs or RCMs) looking at their historical climate, or for driving an RCM. Over the years, the quality of reanalyses has greatly improved, to the point that they are increasingly used as reference data in the statistical downscaling of data from climate models.
To learn more
The “Advancing Reanalysis” website offers information, forums, links to most of the reanalysis databases, and documentation produced by the various institutions.